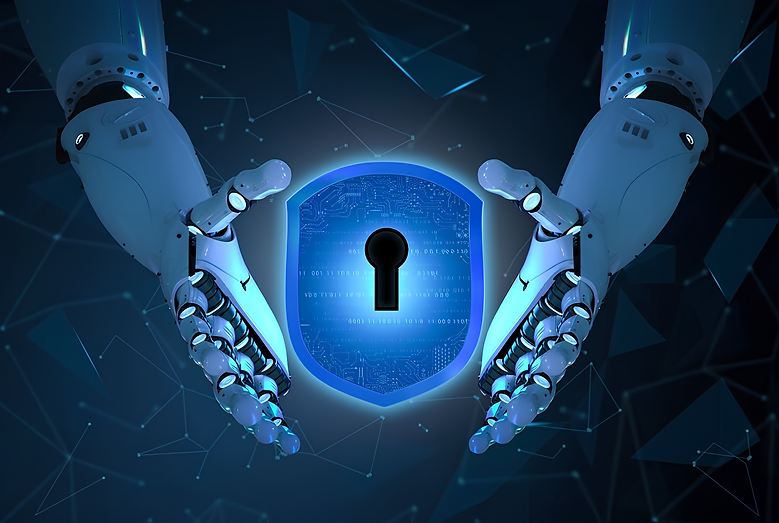
AI Security: The Next Frontier
Safeguard AI systems from threats to ensure reliable and secure operation
75% of security professionals witnessed an increase in attacks over the past 12 months, with 85% attributing this rise to bad actors using generative AI. That means cybercriminals are using Gen AI to create more sophisticated cyber attacks at scale
- Deep Instinct
Generative AI – a New Huge Attack Surface for Enterprises?
Large Language Models (LLMs) like GPT-4 have undeniably revolutionized the field of artificial intelligence with their advanced language processing abilities. However, as these models become more powerful, ensuring their security and privacy has become increasingly crucial. This focus on LLM security arises from the potential risks they pose, including vulnerabilities to malicious attacks, data leaks, and the perpetuation of biased results.
Ensuring the security of large language models is not optional; it is a fundamental requirement to safeguard the privacy and safety of individuals and society as a whole. As these powerful AI systems become increasingly integrated into various aspects of our lives, from communication and education to healthcare and decision-making, the importance of prioritizing their security cannot be overstated.
Studies reveal that despite the widespread adoption of LLMs in the open-source community, the initial projects developed using this technology are largely insecure. Cybersecurity professionals underline the urgent need for improved security standards and practices to mitigate the growing risks in developing and maintaining this technology.
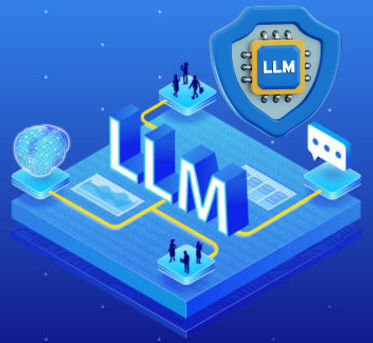
A robust focus on LLM security involves not only preventing potential harm, such as data breaches or malicious use, but also actively shaping a responsible and ethical framework for their development and deployment. This includes addressing concerns related to data privacy, bias in model outputs, and the potential for misuse. By taking proactive steps to mitigate these risks, we can ensure that LLMs are used in a way that benefits humanity while upholding fundamental values of trust, transparency, and accountability.
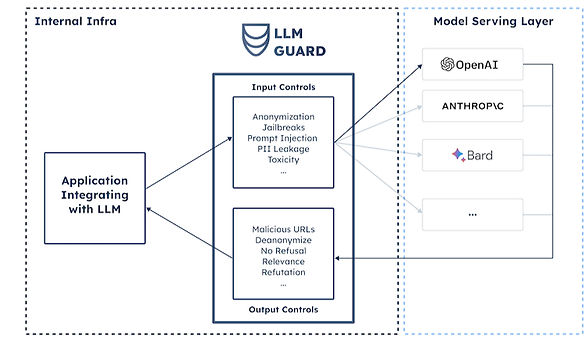
Artificial intelligence is rapidly transforming software applications, but integrating AI models also introduces new risks. As highlighted in a recent analysis by NCC Group, AI-powered apps need an augmented threat model to fully understand and mitigate emerging attack vectors.

New Attack Vectors Targeting AI Systems
The analysis identifies numerous new attack vectors that threat actors could leverage via LLM
-
Prompt injection - Attackers modify model behavior by injecting malicious instructions into prompts. For example, overriding safety clauses.
-
.Oracle attacks - Blackbox models are queried to extract secrets one bit at a time, similar to padding oracle attacks on crypto.
-
Adversarial inputs - Slight perturbations to images, audio or text cause misclassifications. Used to evade spam filters or defeat facial recognition.
-
Format corruption - By manipulating model outputs, attackers can disrupt downstream data consumers that expect structured responses.
-
Water table attacks - Injecting malicious training data skews model behavior. Poisoning attacks have corrupted AI chatbots before.
-
Persistent world corruption - If models maintain state across users, attackers can manipulate that state to impact other users.
-
Glitch tokens - Models mishandle rare or adversarial tokens in unpredictable ways
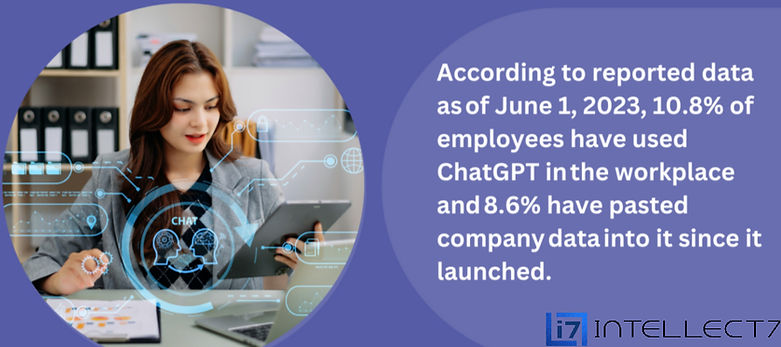

Architecting More Secure AI Applications
To mitigate risks, architects should design security controls around AI models as if they were untrusted components. Key recommendations.
-
Isolate models from confidential data and functionality.
-
Validate and restrict model inputs/outputs strictly.
-
Consider trust boundaries and segment architecture accordingly.
-
Employ compensating controls alongside AI components.

LLM security is like an onion. Each layer must treat the ones outside it as untrusted. To defend against prompt injection the model developer must reason about the user-attacker security boundary, which they are far removed from. It's like balancing sticks stacked end to end.

Audit, Assess Risks and Protect AI-Powered Applications
Discovery and continuous
monitoring of AI inventory
Discover and monitor all models, datasets and prompts and tools used by AI apps in the organization. Detect and mitigate risks, and get full, continuous visibilioty and auditability of the entire app usage.
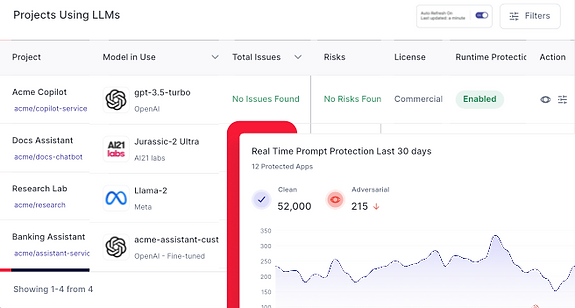
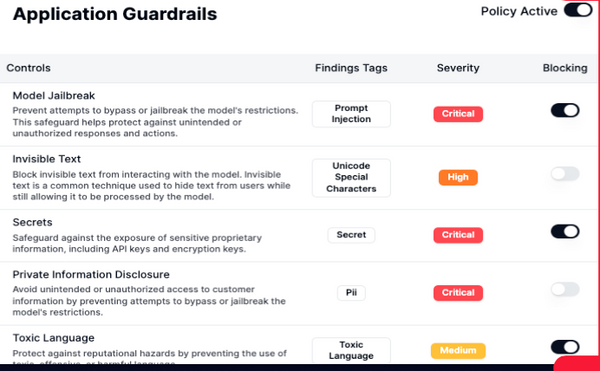
Application-level security controls and policies
Create and enforce data protection, safety and security policies while developing, training or using AI models. Gurantee compliance standards and avoid sensitive data exposure during usage and development.
Create adversarial resistance
to emerging threats
Secure your AI apps from novel risks and vulnerabilities.
Utilize the power of AI without worrying about data leakage, adversarial prompts,, harmful content, safety and other risks,.
